引用本文: | 张勋,黄晓萱,殷金可,陈艳成,林羽,王晓颖,许文.基于近红外和化学计量学方法对金线莲快速鉴别及其多糖含量测定的研究[J].中国现代应用药学,2023,40(19):2702-2712. |
| ZHANG Xun,HUANG Xiaoxuan,YIN Jinke,CHEN Yancheng,LIN Yu,WANG Xiaoying,XU Wen.Rapid Identification and Determination of Polysaccharides Contents in Anoectochilus Roxburghii Based on Near Infrared Spectroscopy with Chemometrics[J].Chin J Mod Appl Pharm(中国现代应用药学),2023,40(19):2702-2712. |
|
|
|
本文已被:浏览 860次 下载 646次 |
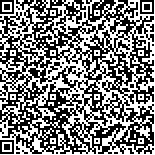 码上扫一扫! |
|
基于近红外和化学计量学方法对金线莲快速鉴别及其多糖含量测定的研究 |
张勋1, 黄晓萱1, 殷金可1, 陈艳成1, 林羽1, 王晓颖1, 许文1,2
|
1.福建中医药大学, 药学院, 福州 350122;2.福建中医药大学, 科技创新与转化中心, 福州 350122
|
|
摘要: |
目的 建立近红外分析方法结合化学计量学算法对金线莲及其近缘品进行判别分析,进一步构建金线莲多糖含量快速预测模型。方法 采集金线莲药材及其近缘品台湾银线兰、血叶兰样品的近红外光谱。以分类准确性为指标优选光谱预处理方法,分别对比决策树、K-近邻算法、随机森林,偏最小二乘回归判别分析、线性判别分析和支持向量机等有监督模式识别算法对样品的分类效果,优选最优算法建立定性模型。应用紫外可见分光光度法结合苯酚硫酸法测定76批金线莲多糖的含量,分别应用支持向量机、极限学习机、决策树、随机森林、主成分回归和偏最小二乘回归等6种不同化学计量学方法关联金线莲多糖含量与近红外光谱,优选定量算法;经光谱预处理和波段选择,基于连续投影算法筛选波段变量数优化并建立金线莲多糖定量模型。结果 以SNV+SG+2ndD为光谱预处理方法结合支持向量机建立的近红外光谱判别分析模型分类准确性最高;基于径向基核函数算法结合混淆矩阵和ROC曲线评价模型的预测性能,模型性能均较优。此外,金线莲近红外原始光谱经SNV+SG+2ndD预处理,以7 000~4 000 cm-1为建模波段,变量数为97,应用连续投影-偏最小二乘构建的定量分析模型准确度较其他算法最高,为0.992。模型的校正集误差均方根为0.625,校正集相关系数为0.993,验证集误差均方根为0.767,验证集相关系数为0.992,预测偏差为8.467,预测集相对偏差<10%。结论 所建立的近红外支持向量机定性和连续投影-偏最小二乘定量模型准确、可靠,可鉴别金线莲真伪以及测定多糖含量,为实现金线莲质量的快速评价提供一种新的方法。 |
关键词: 金线莲 近红外 化学计量学 快速鉴别 多糖含量 |
DOI:10.13748/j.cnki.issn1007-7693.20223221 |
分类号:R284.1 |
基金项目:福建省科技厅高校产学合作项目(2019Y4009);福建省科技厅引导性项目(2017Y0049);福建中医药大学校管课题重点项目(X2021011-重点) |
|
Rapid Identification and Determination of Polysaccharides Contents in Anoectochilus Roxburghii Based on Near Infrared Spectroscopy with Chemometrics |
ZHANG Xun1, HUANG Xiaoxuan1, YIN Jinke1, CHEN Yancheng1, LIN Yu1, WANG Xiaoying1, XU Wen1,2
|
1.Fujian University of Traditional Chinese Medicine, School of Pharmacy, Fuzhou 350122, China;2.Fujian University of Traditional Chinese Medicine, Innovation and Transformation Center of Science and Technology, Fuzhou 350122, China
|
Abstract: |
OBJECTIVE To distinguish Anoectochilus roxburghii and relative species by near infrared(NIR) spectroscopy combined with chemometrics, and to establish a prediction model for rapid determine polysaccharides contents in Anoectochilus roxburghii. METHODS The NIR spectroscopy of Anoectochilus roxburghii, Anoectochilus formosanus Hayata and Ludisia discolor were collected. The prepossessing of original spectrum was optimization through accuracy of classification in the NIR model, and six supervised pattern recognition algorithms such as decision tree, K-nearest neighbor algorithm, random forest, partial least squares regression discriminant analysis, linear discriminant analysis and support vector machine(SVM) were applied to identify effect of the classification effect, optimum algorithm and then establish qualitative model. The content of polysaccharides in 76 batches of Anoectochilus roxburghii samples were examined by ultraviolet visible spectrophotometry combined with phenol sulfuric acid method. In order to select optimization algorithm, six quantitative stoichiometry algorithms consisted of SVM, extreme learning machines, decision trees, random forests, principal component regression and partial least squares regression(PLS) were used to connect polysaccharide content and the NIR spectroscopy in Anoectochilus roxburghii respectively. The best method for determining the content of Anoectochilus roxburghii polysaccharides was further optimized by spectra pretreatment, band selection and number of band variables based on successive projection algorithm(SPA). RESULTS The NIR discriminant analysis model was established by SVM with SNV+SG+2ndD, and the classification accuracy was best. The prediction performance was evaluated based on the radial basis kernel function algorithm combined with confusion matrix and ROC curve, and the model performance was good. In addition, the quantitative analysis model was constructed by continuous projection-partial least squares by the prepossessing of SNV+SG+2ndD and the optimal band of 7 000-4 000 cm-1 with 97 of variables number. The accuracy was 0.992, which was the highest. The root mean square error calibration set, correlation coefficient of calibration set, and the root mean square error in validation set, correlation coefficient of validation set were 0.625, 0.993, 0.767, 0.992, separately. The prediction deviation was 8.467, and relative deviation of prediction set was less than 10%. CONCLUSION The established NIR-SVM qualitative model and SPA-PLS quantitative model are accurate and reliable, which are enable to identify Anoectochilus roxburghii and determine polysaccharide content nondestructively. It is a new and promising method for rapid evaluation of Anoectochilus roxburghii quality. |
Key words: Anoectochilus roxburghii near infrared spectroscopy chemometrics rapid identification polysaccharides contents |
|
|
|
|